일 | 월 | 화 | 수 | 목 | 금 | 토 |
---|---|---|---|---|---|---|
1 | ||||||
2 | 3 | 4 | 5 | 6 | 7 | 8 |
9 | 10 | 11 | 12 | 13 | 14 | 15 |
16 | 17 | 18 | 19 | 20 | 21 | 22 |
23 | 24 | 25 | 26 | 27 | 28 |
Tags
- dann paper
- shrinkmatch
- mme paper
- semi supervised learnin 가정
- dcgan
- UnderstandingDeepLearning
- CoMatch
- 딥러닝손실함수
- ConMatch
- CycleGAN
- conjugate pseudo label paper
- CGAN
- simclrv2
- GAN
- 백준 알고리즘
- cifar100-c
- 컴퓨터구조
- Entropy Minimization
- BYOL
- adamatch paper
- WGAN
- Meta Pseudo Labels
- Pseudo Label
- Pix2Pix
- 최린컴퓨터구조
- remixmatch paper
- mocov3
- shrinkmatch paper
- tent paper
- SSL
Archives
- Today
- Total
목록Positional Encoding (1)
Hello Computer Vision
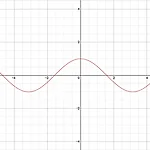
Transformer 구조를 살펴보다 보면 input에 대하여 Embedding을 입힌 후 Positional Encoding하는 과정을 거치는 것을 확인할 수 있다. ViT에서는 안하는 추세이지만 NLP에서는 위치가 중요하다. Positional Encoding 사용 이유 RNN, LSTM 과 다르게 Transformer에서는 가장 큰 특징인 병렬화를 사용하기 때문에 각 단어 토큰에 대한 순서를 보장하지 않는다. 예를 들어 "I do want to buy ice cream, but i can not' 'i do not want to but ice cream, but i can' 방금 급조한 두개의 문장을 비교해본다면 들어간 단어는 똑같으나 단어의 순서에 따라 의미가 확 변하는 것을 확인할 수 있다. 그..
딥러닝
2023. 4. 4. 23:23