일 | 월 | 화 | 수 | 목 | 금 | 토 |
---|---|---|---|---|---|---|
1 | 2 | 3 | 4 | 5 | ||
6 | 7 | 8 | 9 | 10 | 11 | 12 |
13 | 14 | 15 | 16 | 17 | 18 | 19 |
20 | 21 | 22 | 23 | 24 | 25 | 26 |
27 | 28 | 29 | 30 |
- dcgan
- dann paper
- Entropy Minimization
- tent paper
- shrinkmatch paper
- remixmatch paper
- 최린컴퓨터구조
- 백준 알고리즘
- SSL
- 컴퓨터구조
- CoMatch
- shrinkmatch
- cifar100-c
- semi supervised learnin 가정
- mocov3
- Pix2Pix
- conjugate pseudo label paper
- Meta Pseudo Labels
- CGAN
- adamatch paper
- WGAN
- CycleGAN
- ConMatch
- 딥러닝손실함수
- simclrv2
- BYOL
- UnderstandingDeepLearning
- mme paper
- GAN
- Pseudo Label
- Today
- Total
Hello Data
비전공생의 CycleGANCycleconsistentGAN,2017코드 구현 본문
지난 번에 CycleGAN 논문 리뷰를 진행했었는데요, 최근 스터디에서 cycleGAN을 이용해
프로젝트를 진행하려고 해서 빨리 찾아서 공부해봤습니다.
생성자
class Generator(nn.Module):
def __init__(self, img_channels,num_features = 64, num_resblock = 9):
super(Generator, self).__init__()
self.initial = nn.Sequential(
nn.Conv2d(img_channels, num_features, kernel_size = 7, stride = 1, padding = 3, padding_mode = 'reflect'),
nn.ReLU(inplace = True)
)
self.down_blocks = nn.ModuleList(
[
ConvBlock(num_features, num_features * 2, kernel_size = 3, stride = 2, padding = 1),
ConvBlock(num_features * 2, num_features * 4, kernel_size = 3, stride = 2, padding = 1),]
)
self.residual_block = nn.Sequential(
*[ResidualBlock(num_features * 4) for _ in range(num_resblock)]
)
self.up_blocks = nn.ModuleList(
[
ConvBlock(num_features * 4, num_features * 2, down = False, kernel_size = 3, stride = 2,padding = 1, output_padding = 1),
ConvBlock(num_features * 2, num_features, down = False, kernel_size = 3, stride = 2,padding = 1, output_padding = 1)
])
self.last = nn.Conv2d(num_features * 1, img_channels, kernel_size = 7, stride = 1, padding = 3, padding_mode = 'reflect')
def forward(self, x):
x = self.initial(x)
for layer in self.down_blocks:
x = layer(x)
x= self.residual_block(x)
for layer in self.up_blocks:
x = layer(x)
return torch.tanh(self.last(x))
입력받은 이미지에 대해서 downsample을 진행한 후에 upsample을 진행해준다.
지금까지 보통 latent vector에서 이미지를 만드는 과정만 있었기 때문에 생성자에서 upsampling하는 과정만을 거쳤다.
그러나 CycleGAN에서는 이미지를 받고 특징을 다른 이미지로 translation하는 것이 목적이기 때문에
입력받은 이미지의 특징을 압축하는 downsample하고 이미지로 만드는 upsample하는 과정을 거쳤다.
훈련과정은 밑에서 설명하겠습니다.
판별자
class Discriminator(nn.Module):
def __init__(self, in_channels = 3, features = [64, 128, 256, 512]):
super(Discriminator, self).__init__()
self.initial = nn.Sequential(
nn.Conv2d(in_channels, features[0], kernel_size = 4, stride = 2, padding = 1, padding_mode ='reflect'), # Conv(3, 64, 4, 2, 1)
nn.LeakyReLU(0.2))
layers = []
in_channels = features[0]
for feature in features[1:]:
layers.append(Block(in_channels, feature, stride = 1 if feature == features[-1] else 2)) #Conv(64,128, 4, 2, 1),Conv(128,256, 4, 2, 1), Conv(256, 512, 4, 1, 1) 담는다
in_channels = feature #
layers.append(nn.Conv2d(in_channels,1, kernel_size = 4, stride = 1, padding = 1, padding_mode = 'reflect')) #Conv(512, 1, 4, 1, 1)
self.model = nn.Sequential(*layers)
def forward(self, x):
x = self.initial(x)
return torch.sigmoid(self.model(x)) # 30 x 30 pathgan
1개의 값만을 내뱉는 것이 아닌 PatchGAN 을 활용해 30x30을 내뱉을 수 있도록한다.
훈련
disc_H = Discriminator(in_channels = 3).to(device)
disc_Z = Discriminator(in_channels = 3).to(device)
gen_Z = Generator(img_channels = 3, num_resblock = 9).to(device)
gen_H = Generator(img_channels = 3, num_resblock = 9).to(device)
optim_disc = optim.Adam(list(disc_H.parameters()) + list(disc_Z.parameters()),
lr = learning_rate, betas = (0.5, 0.999))
optim_gen = optim.Adam(list(gen_Z.parameters()) + list(gen_H.parameters()),
lr = learning_rate, betas = (0.5, 0.999))
L1 = nn.L1Loss()
mse = nn.MSELoss()
for epoch in range(num_epochs):
for idx, batch in enumerate(dataloader):
orange = batch['B'].to(device)
apple = batch['A'].to(device)
optim_disc.zero_grad()
optim_gen.zero_grad()
fake_apple = gen_A(orange)
D_A_real = disc_A(horse)
D_A_fake = disc_A(fake_horse.detach())
D_A_real_loss = mse(D_A_real, torch.ones_like(D_A_real))
D_A_fake_loss = mse(D_A_fake, torch.zeros_like(D_A_fake))
D_A_loss = D_A_real_loss + D_A_fake_loss
fake_orange = gen_O(apple)
D_O_real = disc_Z(zebra)
D_O_fake = disc_Z(fake_zebra.detach())
D_O_real_loss = mse(D_O_real, torch.ones_like(D_O_real))
D_O_fake_loss = mse(D_O_fake, torch.zeros_like(D_O_fake))
D_O_loss = D_O_real_loss + D_O_fake_loss
D_loss = (D_A_loss + D_O_loss) / 2
D_loss.backward()
optim_disc.step()
#generator 학습
D_A_fake = disc_A(fake_apple)
D_O_fake = disc_O(fake_orange)
loss_G_A = mse(D_Z_fake, torch.ones_like(D_A_fake)) #CE 를 쓰지않고 여기서 정의된 새로운 손실함수
loss_G_O = mse(D_Z_fake, torch.ones_like(D_O_fake))
cycle_orange = gen_O(fake_apple)
cycle_apple = gen_A(fake_orange)
cycle_orange_loss = L1(orange, cycle_orange)
cycle_apple_loss = L1(apple, cycle_apple)
identity_orange = gen_O(orange)
identity_apple = gen_A(apple)
identity_orange_loss = L1(orange, identity_orange)
identity_apple_loss = L1(apple, identity_apple)
G_loss = (loss_G_O + loss_G_A + cycle_orange_loss * lambda_cycle + cycle_apple_loss * lambda_cycle
+identity_apple_loss * lambda_identity + identity_orange_loss * lambda_identity)
G_loss.backward()
optim_gen.step()
if idx == 50:
print('EPOCH : ', epoch, 'G_loss : ', G_loss, 'D_loss :', D_loss)
break
Apple 을 orange로 만드는 G 생성자 훈련위해서는
apple을 생성자에 넣고 이를 orange로 변환하고 싶을 때 판별자는 생성자가 만든 가짜 orange이미지에 대해
진짜 orange와 비교를 하면서 손실값을 알려주며Adversarialloss orange가 나올 수 있도록 노력한다.
그리고 mode collapse 를 없애기 위해 F를 통해 만든 fake apple 이미지를 다시 G에 넣어 orange로 돌리는
loss 를 발생시키면서 cycleconsistencyloss apple 이미지에 대해 더 잘 변환할 수 있도록 loss를 반환시킨다.
F를 훈련시킬 때는 반대로 생각하면 된다.
판별자 훈련의 경우 loss부분이 BCE가 아니며, 각각 G, F가 만든 가짜 이미지에 대해서 진짜를 만들수 있도록
loss를 발생시킵니다.
결과
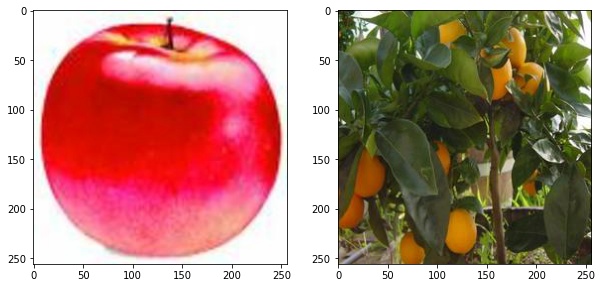
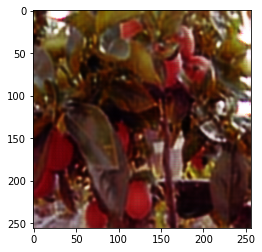
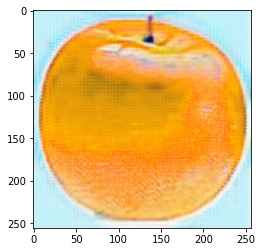
위에 이미지가 원래 이미지이고 밑에 이미지가 G, F생성자를 이용해 만든 이미지이다.
뭔가 그럴듯하게 잘 나온 거 같지만 사과가 오렌지로 변한 것이 아닌 색깔만 변형되었다.
아마 이 부분은 모델이 오렌지의 특징이 노란색인 것을 발견해 이를 입힌 것이 아닐까 싶다.
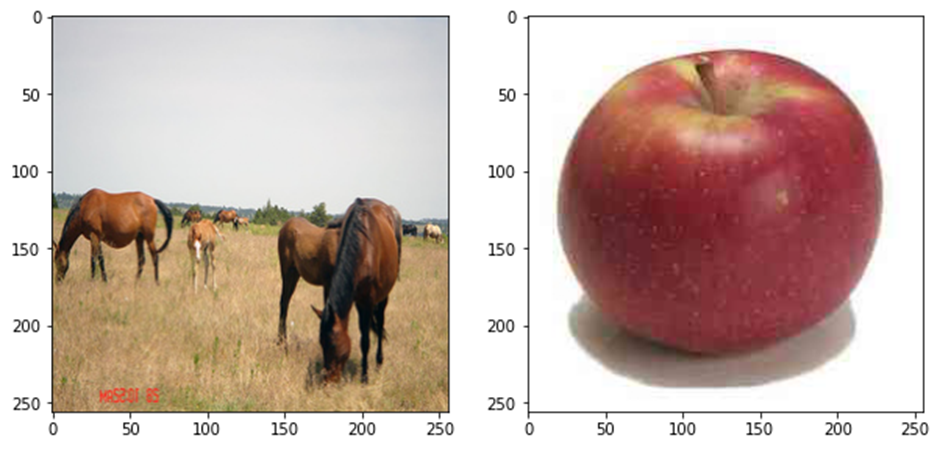
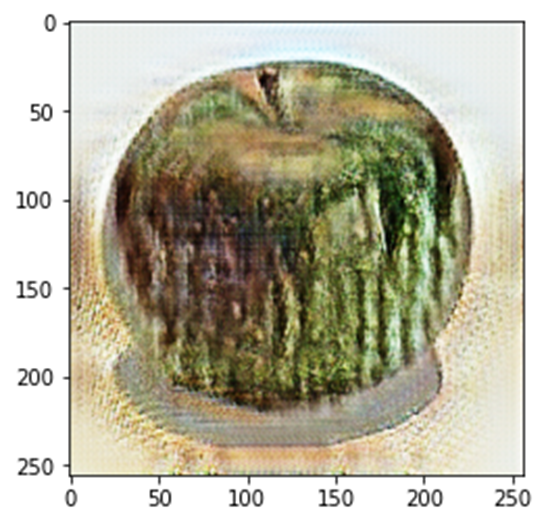
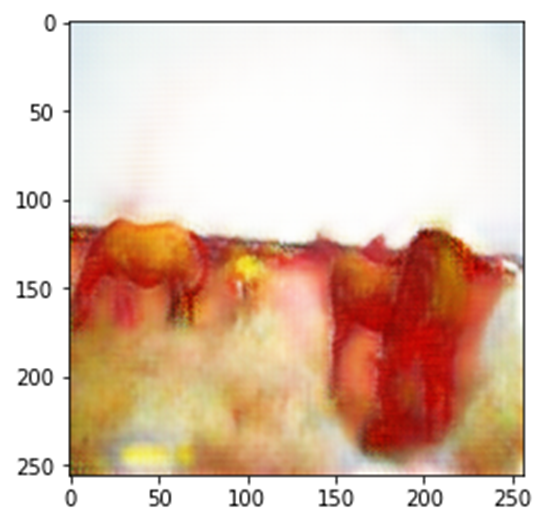
이 결과는 사과와 말을 훈련시킨 모델인데 보시다시피 물체-물체를 훈련시킨다면 색깔 위주로 변형되었음을 확인할 수 있다.
자세히 보면 사과안에 말의 형상이 있음을 확인할 수 있는데 아마 모양같은 디테일은 잘 못바꾸는 거 같다.
전체 코드는 제 깃헙에서 확인할 수 있습니다.
https://github.com/JiWoongCho1/Gernerative-Model_Paper_Review/blob/main/CycleGAN%202.ipynb
GitHub - JiWoongCho1/Gernerative-Model_Paper_Review: 생성모델 논문 review 및 코드 구현입니다.
생성모델 논문 review 및 코드 구현입니다. Contribute to JiWoongCho1/Gernerative-Model_Paper_Review development by creating an account on GitHub.
github.com